Representing higher-order dependencies in networks
To ensure the correctness of network analysis methods, the network (as the input) has to be a sufficiently accurate representation of the underlying data. However, when representing sequential data from complex systems, such as global shipping traffic or Web clickstream traffic as networks, conventional network representations that implicitly assume the Markov property (first-order dependency) can quickly become limiting. This assumption holds that, when movements are simulated on the network, the next movement depends only on the current node, discounting the fact that the movement may depend on several previous steps. However, we show that data derived from many complex systems can show up to fifth-order dependencies. In these cases, the oversimplifying assumption of the first-order network representation can lead to inaccurate network analysis results. To address this problem, we propose the higher-order network (HON) representation that can discover and embed variable orders of dependencies in a network representation. Through a comprehensive empirical evaluation and analysis, we establish several desirable characteristics of HON, including accuracy, scalability, and direct compatibility with the existing suite of network analysis methods. We illustrate how HON can be applied to a broad variety of tasks, such as random walking, clustering, and ranking, and we demonstrate that, by using it as input, HON yields more accurate results without any modification to these tasks.
Cite as: Jian Xu, Thanuka L. Wickramarathne, and Nitesh V. Chawla. "Representing higher-order dependencies in networks." Science Advances 2, no. 5 (2016): e1600028.
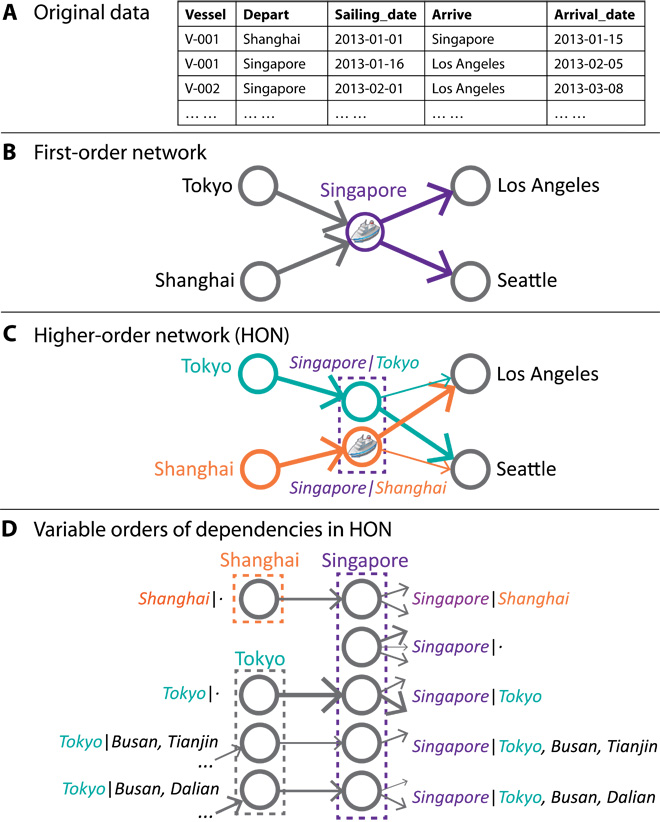
HoNVis: Visualizing and Exploring Higher-Order Networks
Unlike the conventional first-order network (FoN), the higher-order network (HoN) provides a more accurate description of transitions by creating additional nodes to encode higher-order dependencies. However, there exists no visualization and exploration tool for the HoN. For applications such as the development of strategies to control species invasion through global shipping which is known to exhibit higher-order dependencies, the existing FoN visualization tools are limited. In this paper, we present HoNVis, a novel visual analytics framework for exploring higher-order dependencies of the global ocean shipping network. Our framework leverages coordinated multiple views to reveal the network structure at three levels of detail (i.e., the global, local, and individual port levels). Users can quickly identify ports of interest at the global level and specify a port to investigate its higher-order nodes at the individual port level. Investigating a larger-scale impact is enabled through the exploration of HoN at the local level. Using the global ocean shipping network data, we demonstrate the effectiveness of our approach with a real-world use case conducted by domain experts specializing in species invasion. Finally, we discuss the generalizability of this framework to other real-world applications such as information diffusion in social networks and epidemic spreading through air transportation.
Cite as: Jun Tao, Jian Xu, Chaoli Wang, and Nitesh V. Chawla. "HoNVis: Visualizing and Exploring Higher-Order Networks". IEEE PacificVis, 2017
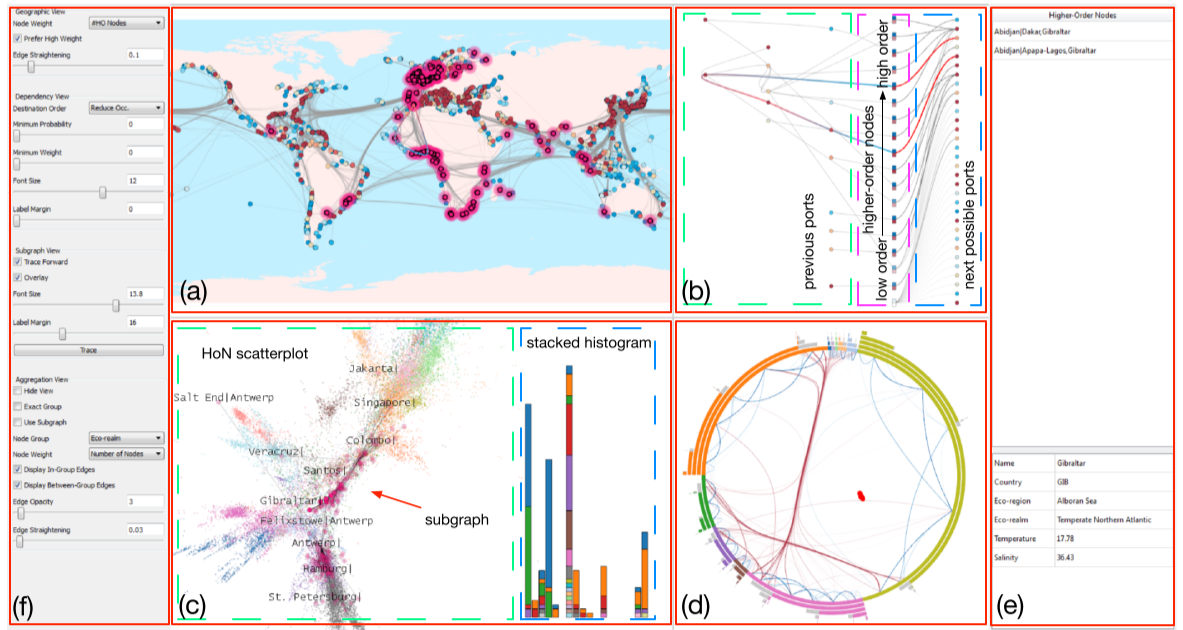
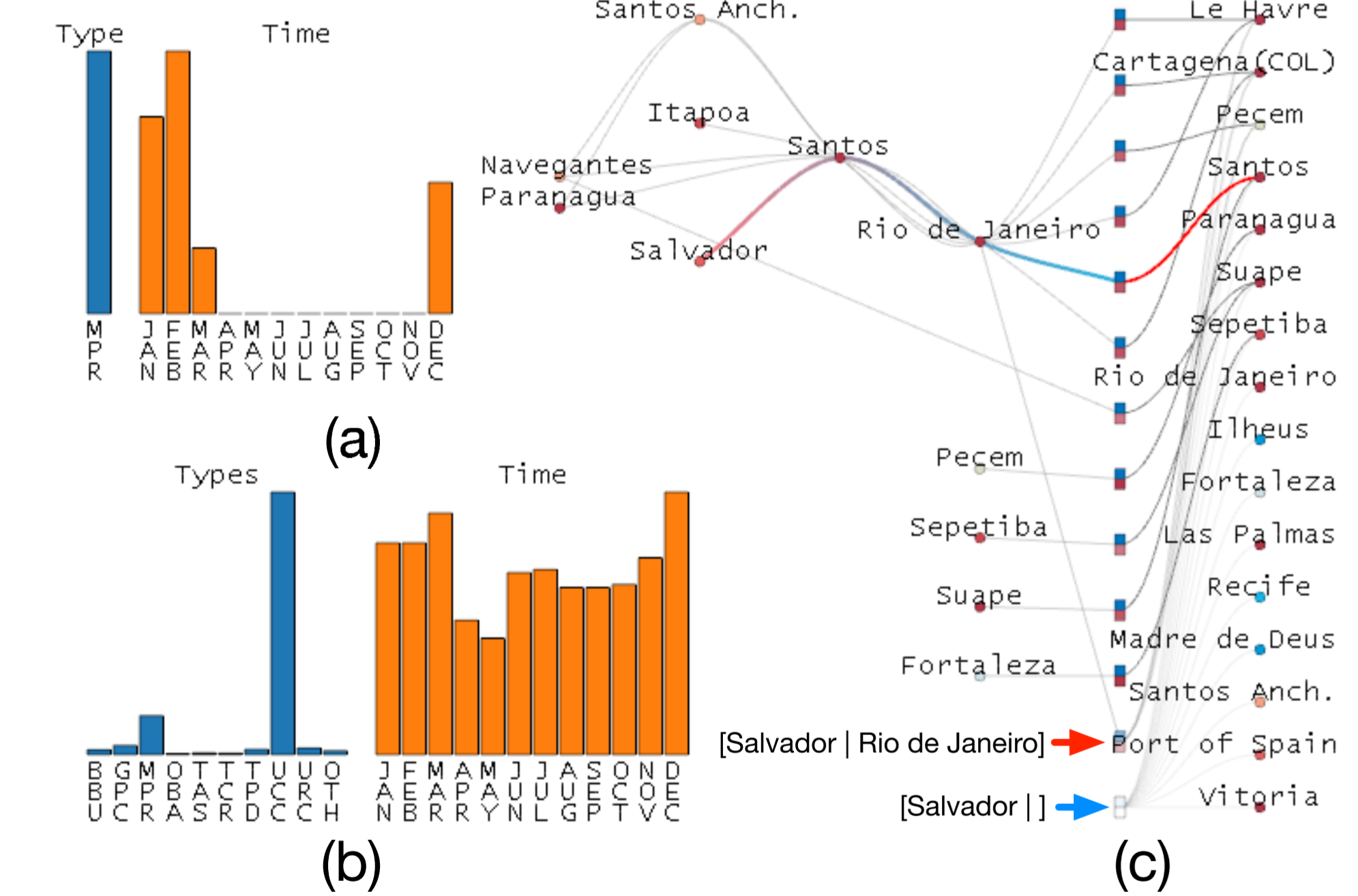
Efficient modeling of higher-order dependencies in networks: from algorithm to application for anomaly detection
Complex systems, represented as dynamic networks, comprise of components that influence each other via direct and/or indirect interactions. Recent research has shown the importance of using Higher-Order Networks (HONs) for modeling and analyzing such complex systems, as the typical Markovian assumption in developing the First Order Network (FON) can be limiting. This higher-order network representation not only creates a more accurate representation of the underlying complex system, but also leads to more accurate network analysis. In this paper, we first present a scalable and accurate model, BuildHON+, for higher-order network representation of data derived from a complex system with various orders of dependencies. Then, we show that this higher-order network representation modeled by BuildHON+ is significantly more accurate in identifying anomalies than FON, demonstrating a need for the higher-order network representation and modeling of complex systems for deriving meaningful conclusions.
Cite as: Saebi, M., Xu, J., Kaplan, L. M., Ribeiro, B., & Chawla, N. V. (2020). Efficient modeling of higher-order dependencies in networks: from algorithm to application for anomaly detection. EPJ Data Science, 9(1), 15.
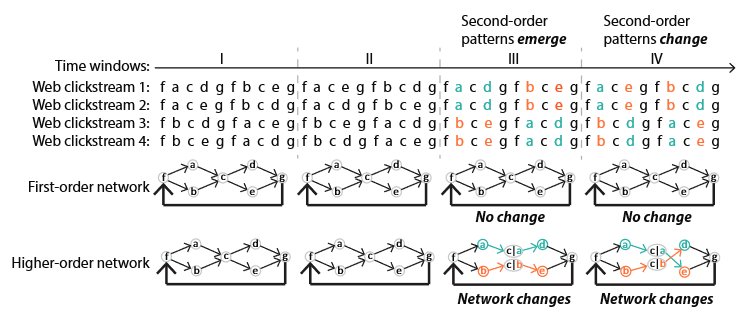
Higher-order patterns of aquatic species spread through the global shipping network
The introduction and establishment of nonindigenous species (NIS) through global ship movements poses a significant threat to marine ecosystems and economies. While ballast-vectored invasions have been partly addressed by some national policies and an international agreement regulating the concentrations of organisms in ballast water, biofouling-vectored invasions remain largely unaddressed. Development of additional efficient and cost-effective ship-borne NIS policies requires an accurate estimation of NIS spread risk from both ballast water and biofouling. We demonstrate that the first-order Markovian assumption limits accurate modeling of NIS spread risks through the global shipping network. In contrast, we show that higher-order patterns provide more accurate NIS spread risk estimates by revealing indirect pathways of NIS transfer using Species Flow Higher-Order Networks (SF-HON). Using the largest available datasets of non-indigenous species for Europe and the United States, we then compare SF-HON model predictions against those from networks that consider only first-order connections and those that consider all possible indirect connections without consideration of their significance. We show that not only SF-HONs yield more accurate NIS spread risk predictions, but there are important differences in NIS spread via the ballast and biofouling vectors. Our work provides information that policymakers can use to develop more efficient and targeted prevention strategies for ship-borne NIS spread management, especially as management of biofouling is of increasing concern.
Cite as: Saebi, M., Xu, J., Grey, E. K., Lodge, D. M., Corbett, J. J., & Chawla, N. (2020). Higher-order patterns of aquatic species spread through the global shipping network. Plos one, 15(7), e0220353.
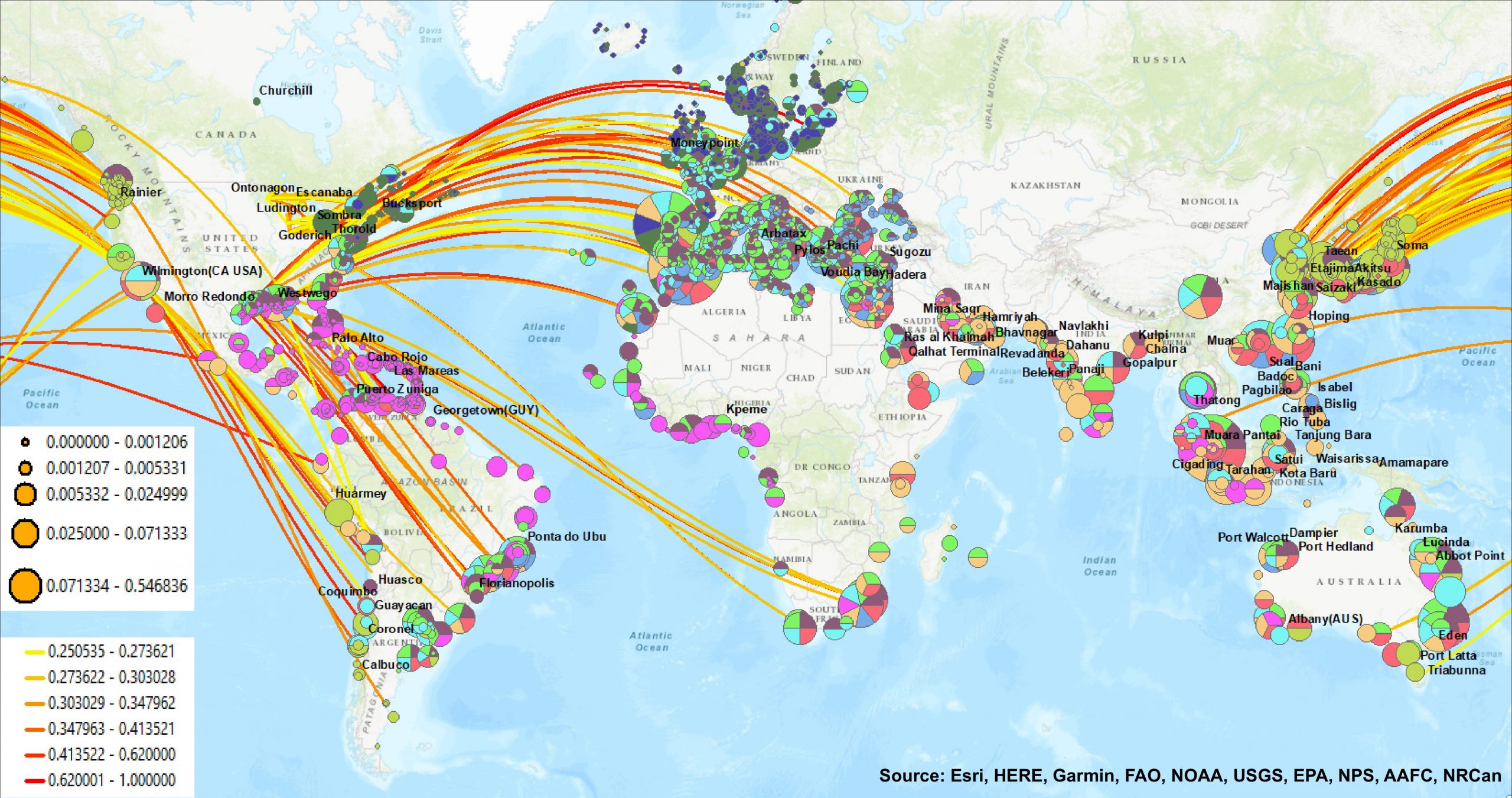
HONEM: Learning Embedding for Higher Order Networks
Representation learning on networks offers a powerful alternative to the oft painstaking process of manual feature engineering, and, as a result, has enjoyed considerable success in recent years. However, all the existing representation learning methods are based on the first-order network, that is, the network that only captures the pairwise interactions between the nodes. As a result, these methods may fail to incorporate non-Markovian higher order dependencies in the network. Thus, the embeddings that are generated may not accurately represent the underlying phenomena in a network, resulting in inferior performance in different inductive or transductive learning tasks. To address this challenge, this study presents higher order network embedding (HONEM), a higher order network (HON) embedding method that captures the non-Markovian higher order dependencies in a network. HONEM is specifically designed for the HON structure and outperforms other state-of-the-art methods in node classification, network reconstruction, link prediction, and visualization for networks that contain non-Markovian higher order dependencies.
Cite as: Saebi, M., Ciampaglia, G. L., Kaplan, L. M., & Chawla, N. V. (2020). HONEM: Learning Embedding for Higher Order Networks. Big Data, 8(4), 255-269.
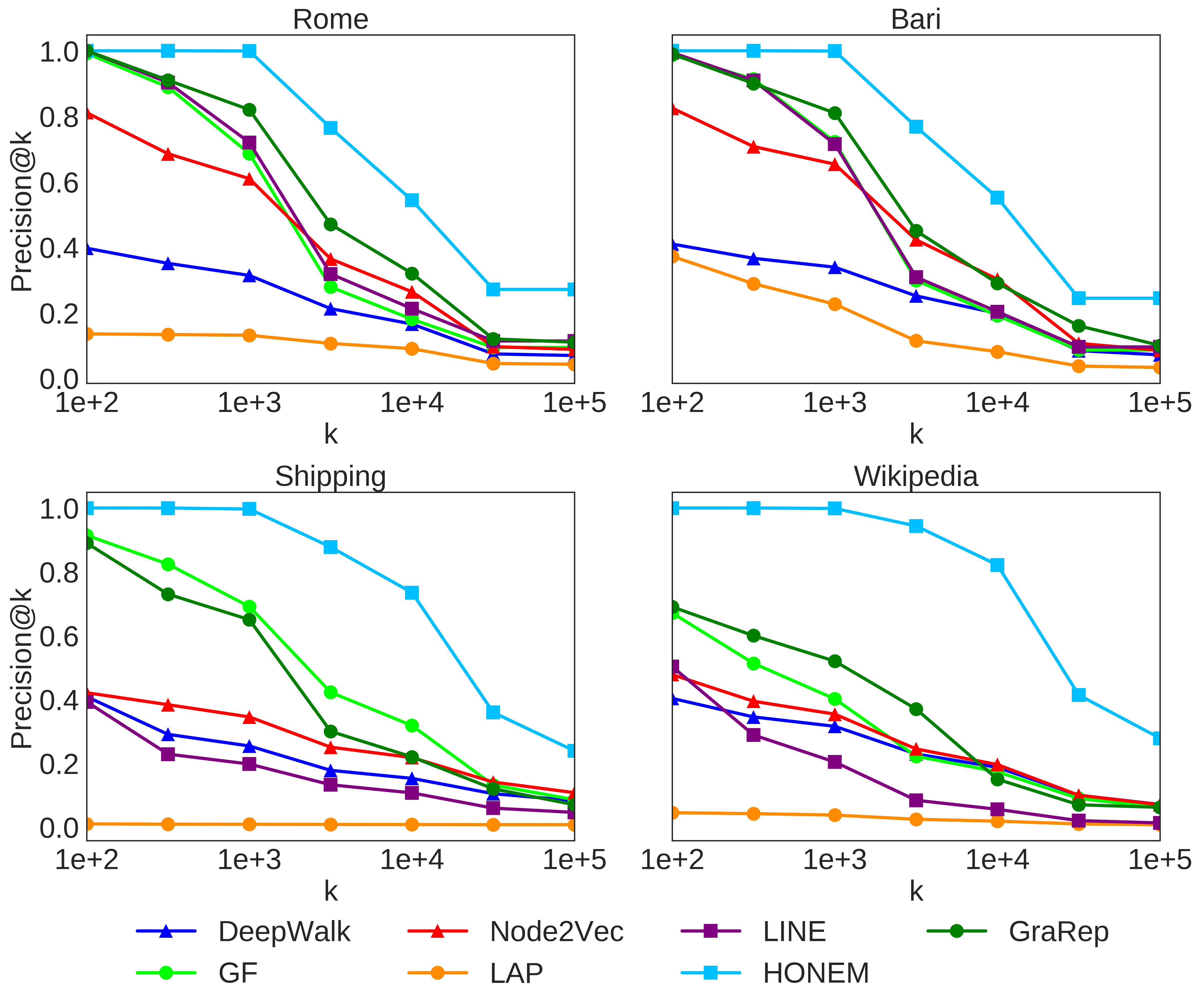
Network analysis of ballast-mediated species transfer reveals important introduction and dispersal patterns in the Arctic
Rapid climate change has wide-ranging implications for the Arctic region, including sea ice loss, increased geopolitical attention, and expanding economic activity, including a dramatic increase in shipping activity. As a result, the risk of harmful non-native marine species being introduced into this critical region will increase unless policy and management steps are implemented in response. Using big data about shipping, ecoregions, and environmental conditions, we leverage network analysis and data mining techniques to assess, visualize, and project ballast water-mediated species introductions into the Arctic and dispersal of non-native species within the Arctic. We first identify high-risk connections between the Arctic and non-Arctic ports that could be sources of non-native species over 15 years (1997-2012) and observe the emergence of shipping hubs in the Arctic where the cumulative risk of non-native species introduction is increasing. We then consider how environmental conditions can constrain this Arctic introduction network for species with different physiological limits, thus providing a species-level tool for decision-makers. Next, we focus on within-Arctic ballast-mediated species dispersal where we use higher-order network analysis to identify critical shipping routes that may facilitate species dispersal within the Arctic. The risk assessment and projection framework we propose could inform risk-based assessment and management of ship-borne invasive species in the Arctic.
Cite as: Saebi, M., Xu, J., Curasi, S. R., Grey, E. K., Chawla, N. V., & Lodge, D. M. (2020). Network analysis of ballast-mediated species transfer reveals important introduction and dispersal patterns in the Arctic. arXiv preprint arXiv:2009.12728.
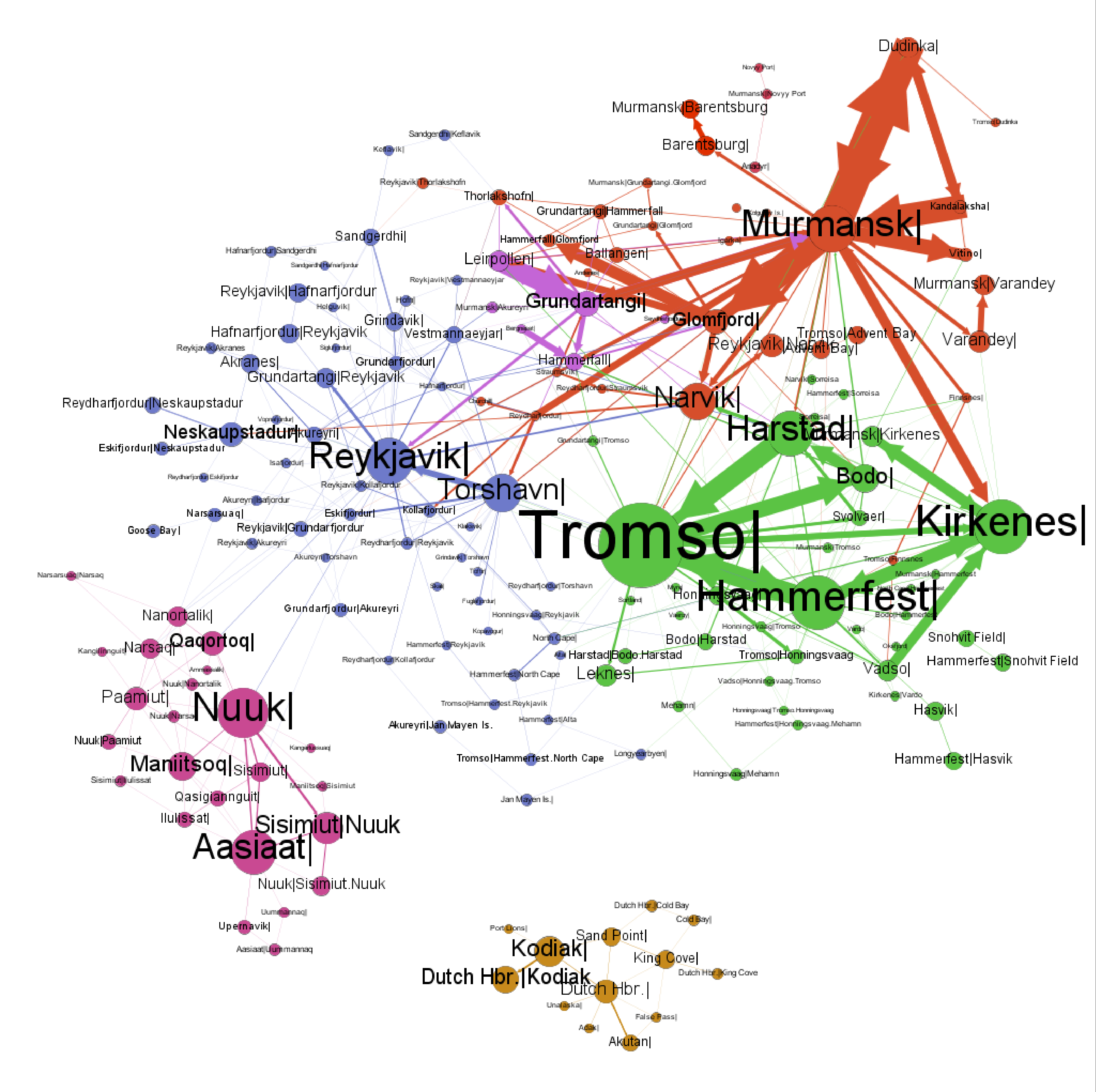
In the news
Complex inter-dependent data: Looking beyond conventional networks can lead to better predictions, by ScienceDaily
New research suggests that current algorithms to represent networks have not truly considered the complex inter-dependencies in data, which can lead to erroneous analysis or predictions. Scientists have now developed a new algorithm that offers the promise of more precise network representation and accurate analysis.... Click to view more
We're Modeling Networks All Wrong, by Motherboard, Vice
Current network analysis algorithms are missing or skipping over some crucial details, argues a team of computer science researchers from the University of Notre Dame in the current issue of Science Advances. The result, they say, is a widespread pattern of oversimplification profound enough to lead to erroneous results and interpretations. Naturally, the scientists have developed a new and improved alternative capable of spotting missed interdependencies... Click to view more